A Collaborative Framework Using Multimodal Data and Adaptive Noise for Human Behavior Anomaly Detection
Jun 30, 2024·,
,,,·
0 min read
Guoqing Yang
Jianzhe Gao

Kejia Zhang
Yifan He
Zhiming Luo
Shaozi Li
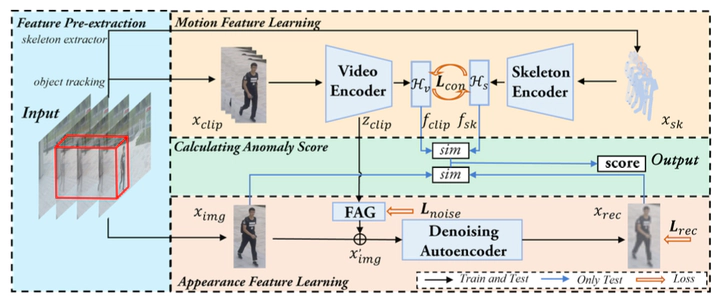
Abstract
Human behavior anomaly detection in video aims to identify unusual behaviors that are crucial for public safety. Re- cently, there has been an increase in reconstruction or prediction- based methods that integrate diverse modal features to enhance anomaly detection. However, they use methods that independently or directly fusion multimodal features without fully considering the collaborative potential between multimodal features, which are susceptible to interference from semantic differences, thereby impacting detection performance. In contrast, we design a collaborative framework using multimodal data and adaptive noise for behavior anomaly detection. Our framework detects anomalies by analyzing the contrastive differences between two modalities alongside single-frame reconstruction errors. Specif- ically, we first learn the correlation between RGB and skeletal modalities for normal behavior through contrastive learning and use inter-modal contrast difference to detect motion anomalies. Additionally, we propose a single-frame reconstruction network that adaptively adds noise based on the importance of foreground features to detect appearance anomalies. Anomalies often occur in the motion foreground, and increasing noise in this area can make it more difficult to reconstruct anomalies. Extensive experiments validate the state-of-the-art performance of our method on three public datasets.
Type
Publication
2024 International Joint Conference on Neural Networks (IJCNN)