Mitigating Low-Frequency Bias: Feature Recalibration and Frequency Attention Regularization for Adversarial Robustness
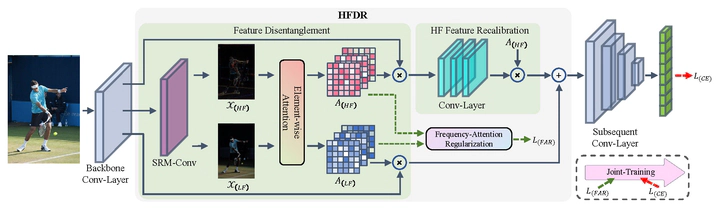
Abstract
Ensuring the robustness of deep neural networks against adversarial attacks remains a fundamental challenge in computer vision. While adversarial training (AT) has emerged as a promising defense strategy, our analysis reveals a critical limitation:AT-trained models exhibit a bias toward low-frequency features while neglecting high-frequency components. This bias is particularly concerning as each frequency component carries distinct and crucial information:low-frequency features encode fundamental structural patterns, while high-frequency features capture intricate details and textures. To address this limitation, we propose High-Frequency Feature Disentanglement and Recalibration (HFDR), a novel module that strategically separates and recalibrates frequency-specific features to capture latent semantic cues. We further introduce frequency attention regularization to harmonize feature extraction across the frequency spectrum and mitigate the inherent low-frequency bias of AT. Extensive experiments demonstrate our method’s superior performance against white-box attacks and transfer attacks, while exhibiting strong generalization capabilities across diverse scenarios.
Type
Publication
arxiv